Upcoming Events
CSE Faculty Candidate Seminar - Ming Yin
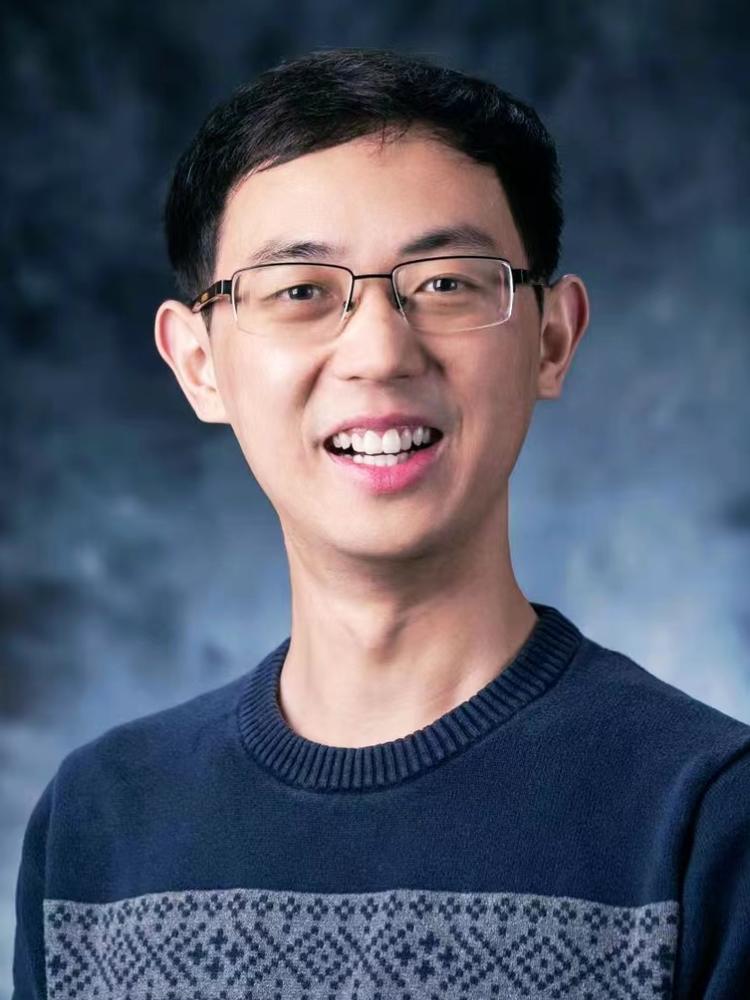
Name: Ming Yin, Postdoctoral Associate from Princeton University
Date: Thursday, February 6, 2024 at 11:00 am
Location: Scheller College of Business, Room 200 (Google Maps link)
Link: The recording of this in-person seminar will be uploaded to CSE's MediaSpace
Coffee, drinks, and snacks provided!
Title: Reinforcement Learning at the Frontier: From Better AI Models to the Acceleration of Scientific Discovery
Abstract: A central challenge in AI is steering complex systems toward desired outcomes—whether in decision-making, aligning language models, or driving scientific discovery. As AI scales with big data and foundation models, reinforcement learning (RL) has emerged as a powerful framework for optimizing behavior through interaction and feedback. In this talk, I will present my research at the intersection of Reinforcement Learning and Artificial Intelligence. First, I will discuss my theoretical contributions that improve the existing understanding of foundational RL, providing optimal guarantees and practical insights. Building on this, I will introduce how RL principles underpin LLM alignment, illustrating how decoding can be framed as a policy optimization problem. Our method significantly enhances inference-time alignment, demonstrating state-of-the-art improvements validated by various metrics. Moving beyond language models, I will highlight CRISPR-GPT—an AI agent that redefines precision in gene-editing research by integrating domain expertise, validated libraries, and computational tools. CRISPR-GPT automates end-to-end gene-editing workflows and, in a real-world case study, achieved 80% editing efficiency in A549 cells, significantly accelerating lung cancer research by eliminating months of trial-and-error. By bridging theory and practice, this talk aspires to spark a new wave of AI research that amplifies scientific exploration and societal benefits.
Bio: Ming Yin is a postdoctoral associate in the Electrical and Computer Engineering Department at Princeton University. He holds dual PhDs in Computer Science and Statistics from the University of California, Santa Barbara. His research spans the theory, algorithms, and applications of machine learning and artificial intelligence, with a particular emphasis on reinforcement learning and generative AI. His work has been published in top venues such as NeurIPS, ICML, and ICLR, and with an oral presentation at AISTATS. Ming’s contributions have earned multiple Rising Star Awards and recognition as a Best Paper Finalist at CVPR 2024. He has served as a senior program committee member for many leading machine learning conferences and as an area chair for NeurIPS and ICML. Beyond academia, he gained industry experience through two summers at Amazon AWS AI.
Event Details
Media Contact
Mary High
mhigh7@gatech.edu
EVENTS BY SCHOOL & CENTER
School of Computational Science and Engineering
School of Interactive Computing
School of Cybersecurity and Privacy
Algorithms and Randomness Center (ARC)
Center for 21st Century Universities (C21U)
Center for Deliberate Innovation (CDI)
Center for Experimental Research in Computer Systems (CERCS)
Center for Research into Novel Computing Hierarchies (CRNCH)
Constellations Center for Equity in Computing
Institute for People and Technology (IPAT)
Institute for Robotics and Intelligent Machines (IRIM)